Observing Learning Through the Lenses of Big Data
Learning is a fascinatingly complicated behavior. Every student learns at a different pace, utilizes different cognitive abilities, displays unique strengths, weaknesses, habits and styles. Yet on any traditional exam, this extremely rich and complicated behavior is often reflected by just one number, most of the time without units.
Online education and big data provides us with a powerful lens capable of observing the dynamics of learning in a large student population at unprecedented resolution. However, data collected from many online courses that are modeled after the traditional lecture-homework-quiz model are so noisy and difficult to understand that it often reminds me of the first batch of photographs taken by the Hubble space telescope. [1]
Collaborating with the Center for Distributed Learning at UCF, I am developing a series of new online learning modules optimized for data collection and frequent learning measurement. Data collected from those online modules is starting to reveal the rich and complex patterns in student learning behavior, and creating exciting new opportunities for education research and educational data mining.
Related talks and publications:
AAPT 2019
Comparing student behavior in mastery and conventional style online physics homework
Earlier
2017 Summer AAPT Talk (Cincinnati)
2017 Summer PERC Poster (Cincinnati)
Creating a “living” Course: Modularized Online Instructional Design
Many say that the traditional lecture based courses are dead. I disagree. It never had a life to begin with. Living organisms, or at least most multi-cellular organisms, display several common features: They are made up of individual living cells, they are good at sensing the surroundings and respond to changes. More importantly, they continue to evolve and adapt to the environment. The traditional course feels more like a brick: it is comes as a whole piece, has a fixed size with stagnant content, and can barely sense the students interacting with it.
By reviving the idea of modularized instructional design which dates back to as early as the 1970s, and combining it with online learning technology, I am trying to build a “living” course, one cell at a time, giving it the ability to continuously evolve and adapt to the learning needs of students today.
Selected Publications
[1] Lee S, Chen Z, Pritchard D, Kimn A and Paul A Factor Analysis Reveals Student Thinking using the Mechanics Reasoning Inventory Proceedings of the Fourth (2017) ACM Conference on Learning @ Scale – L@S ’17 (New York, New York, USA: ACM Press) pp 197–200
[3] Alexandron G, Ruiperez-Valiente J A, Chen Z, Pedro J. Muñoz-Merino and Pritchard D E Copying @ Scale: Using Harvesting Accounts for Collecting Correct Answers in a MOOC Comput. Educ. 108
Selected Talk Videos
2014 Learning with MOOCs conference: Researching for better instructional methods using AB testing
Biography
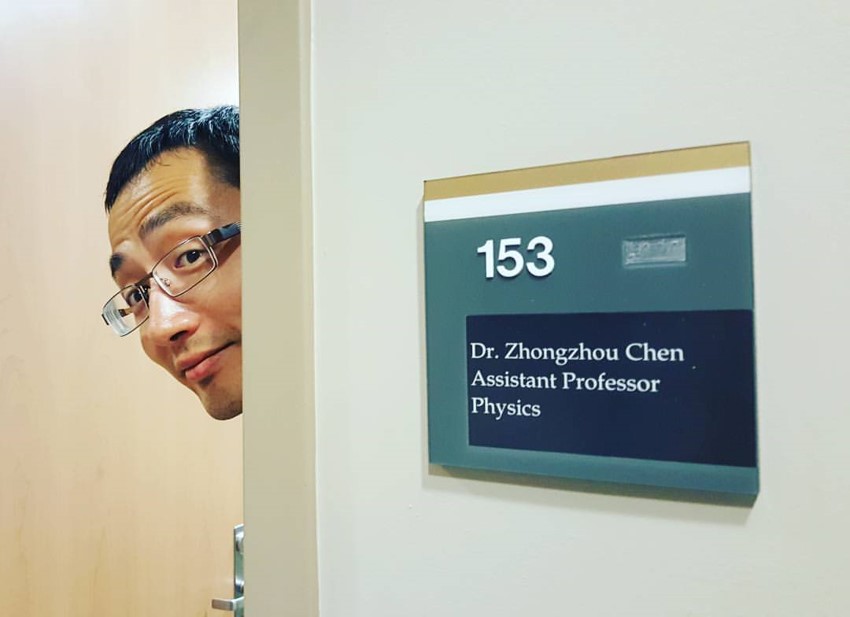
Dr. Zhongzhou Chen (commonly referred to as “Chen” because “Zhongzhou” is just too hard to pronounce in English) is a physics education researcher enthusiastic about re-imagining and reshaping physics and STEM education using online instructional technologies and cognitive science.
Dr. Chen obtained his Ph.D in physics from University of Illinois at Urbana Champaign, studying how Grounded Cognition can be applied to improve the design of instructional animations in physics. He worked as a postdoctorate research assistant at MIT for three years, being mentored (and significantly influenced) by Dave Pritchard.
Early Career:
Chen was born in Shanghai, China, where he obtained his B.S. degree as an illumination engineer from Fudan University. As an undergraduate, he spent most his time working on theoretical computation of Vortex Lattice in Bose-Einstein Condensate, which has nothing to do with illumination. He came to the United States in 2005 as a graduate student and worked for two years in the Computational Biophysics Group lead by the late Klaus Schulten, studying molecular simulation of bacteria flagellum, which clearly has nothing to do with either Bose-Einstein Condensate or Illumination Engineering. After that, he decided to switch from studying bacteria locomotion to understanding how student learns physics.
Contact:
Address: 4111 Libra Drive, PSB 153 Orlando, FL 32816
E-mail: Zhongzhou.Chen@ucf.edu
Life:
Chinese Stand-up Comedy (相声): My youtube 相声 channel
My biggest bass caught since moving to Orlando:

Basketball: I arrived at UIUC the same year Deron Williams graduated, moved to Boston immediately after the Celtics traded Kevin Garnet and Paul Pierce, was rejected by a University in San Francisco in 2016, and now I’m in Orlando. Sorry Magic Fans.