Statistics and Data Science Team Wins Third Consecutive First Place in Competition
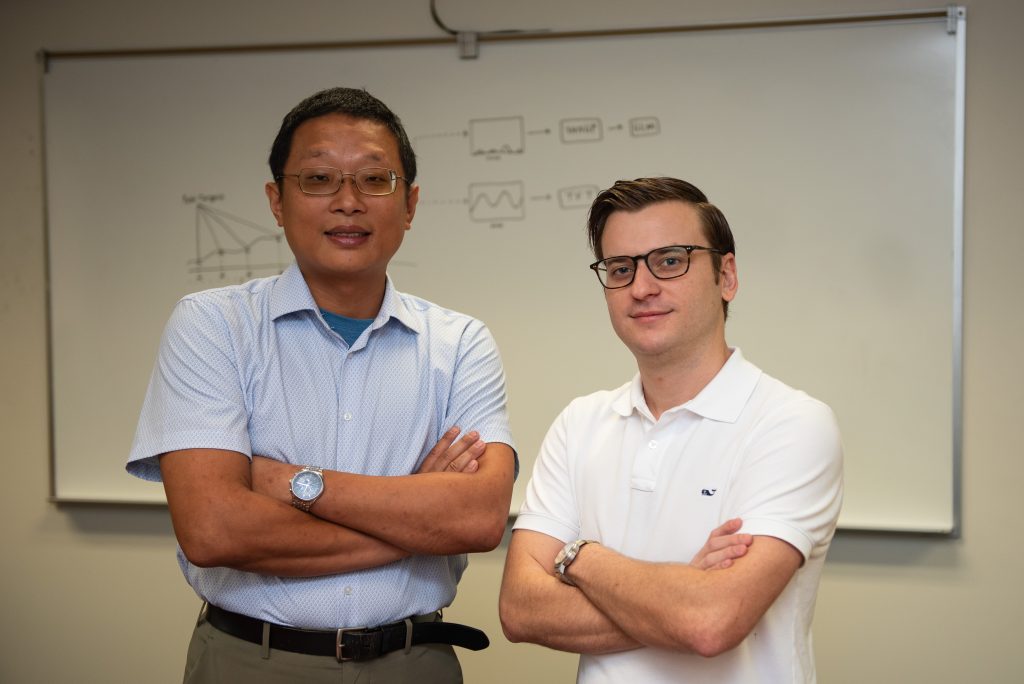
Outbreaks of violence and instability in global hotspots like the Middle East can seem unpredictable, but data science proves otherwise.
That was the premise behind a statistics and deep learning hybrid model that earned Associate Professor Hsin-Hsiung “Bill” Huang, Ph.D., and a team of students from the Department of Statistics and Data Science their third consecutive first place finish in the Algorithms for Threat Detection (ADT) Challenge competitions.
The challenge issued by ADT in 2023 was to map the weekly “events” from 2014 to 2020 within a 21-country region broadly bounded by the Middle East. The 20 event types — as defined by the CAMEO Coding System — span significant escalation like “fight” and “exhibit military posture” to more nuanced events like “engage in diplomatic cooperation.” These real events are recorded by the GDELT Project.
It is then up to the teams to interpret and develop an algorithm using Bayesian spatial and temporal statistical models and deep learning time series modeling that shows the big picture over time. Competition rules limited the time for running the simulation to four hours and the processing power of the computers.
After running their models for predicting more than 6,000 time series simultaneously, the UCF team led by Huang produced an algorithm that predicts events occurring in different regions over time. The finished product correctly matched with real events. For instance, spikes in the time series for “engage in diplomatic cooperation” corresponded with President Donald Trump recognizing Jerusalem as the capital of Israel in 2017. There was a spike in 2018 that coincided with the “great march of return” protests in Gaza.
Evaluation of the models is measured by the lowest percentage of error out of all the simulations. UCF recorded winning the most time units for all event types and the overall lowest error rate.
Joining Huang on the winning team are Hayden Hampton and Chandra Kundu, doctoral candidates in the Department of Statistics and Data Science.
The natural extension of this research is forecasting. While statistical learning is not a crystal ball, it can sift through random events and pick out trends. The federal government — represented at ADT by the Division of Mathematical Sciences (DMS) at the National Science Foundation (NSF) and the National Geospatial Intelligence Agency (NGA) — takes a keen interest in anticipating global unrest. Further refining and advancing the algorithm holds high potential for global security. Huang was awarded the NSF ATD grants in 2019 and 2023.
For Huang, the value of the work is matched by the joy of using his talents in data science to bring order to chaos.
“While some people see randomness, our algorithm shows patterns,” he said.