The University of Central Florida, Department of Mathematics:
Research Experience for Undergraduates in Applied and Computational Mathematics for Summer 2024
Principle Investigator (PI): Dr. Katiuscia Teixeira
Faculty Mentors: Prof. Eduardo Teixeira, Dr. Carlos Borges, Dr. Yukun Li, Dr. Zhisheng Shuai, Dr. Katiuscia Teixeira
Program Dates: May 20 to July 26, 2024
Application Deadline: March 1, 2024 at 11:59 PM
Award Notification: March 27, 2024
Applications for the REU in Applied & Computational Mathematics are Closed
The Research Experience for Undergraduates (REU) in Applied and Computational Mathematics (ACM) 2024 program is a 10-week intensive program that actively engages undergraduate students in research-based theory and applications of mathematical technologies supporting new solutions to interdisciplinary problems.
The program will be held in person from May 20 through July 26, 2024 at the University of Central Florida, located in Orlando, Florida.
Students will work full-time on their individual or in group research projects during the summer semester. The research projects aim to address important questions in applied partial differential equations, computational mathematics, financial engineering, and mathematics biology.
The REU participants’ activities will include short courses on math contents and software, weekly research group meetings, seminars on ethics, discrimination and harassment, career opportunities, and preparation for graduate school, and social events.
Application Requirements
The student application packet consists of the completion of an online form and the submission of
- CV/Resume
- Unofficial Transcripts
- Letter of interest
- Contact information for two people who can provide a Letter of Recommendation for you (email is sufficient)
Eligibility
The program is open to
- U.S. Citizens or permanent residents of the United States;
- Sophomores, juniors, and seniors majoring in
- Pure or applied mathematics,
- Physics
- Computer sciences
- Engineering
- GPA of 2.7 or above;
- Successfully completed Calculus II;
Applications from women and underrepresented minority students are strongly encouraged.
Stipend and Commitment
Each student will receive:
- $6,000 for the summer
- Small food allowance
- On-campus housing
- Students must be willing to live in group housing. For more information, please visit the UCF Housing website.
Faculty Mentors and Research Areas
Project 1: Partial Differential Equations
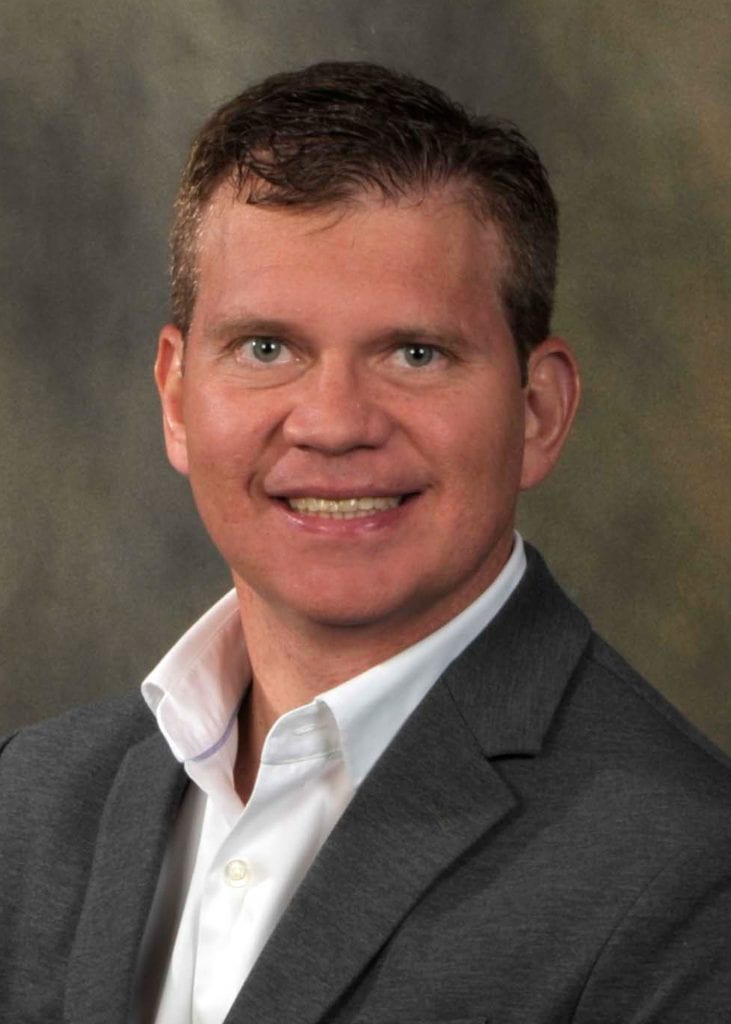
Faculty Mentor:
Prof. Eduardo Teixeira
His research revolves around regularity theory for diffusion PDEs as well as free boundary problems. In the summer of 2024, REU students will be working on projects related to calculus of variations and optimal design problems.
Project 2: Computational Mathematics
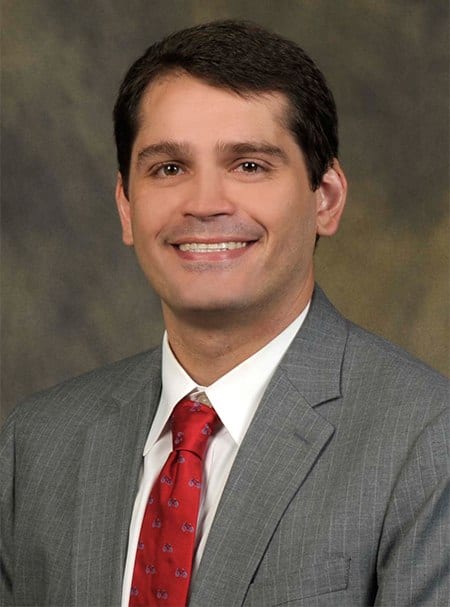
Faculty Mentor:
Dr. Carlos Borges
His research focuses on computational methods for inverse problems. In the Summer of 2024, REU students will be working on projects related to the modeling and development of fast and accurate solvers for the solution of forward and inverse scattering problems.
Project 3: Financial Mathematics
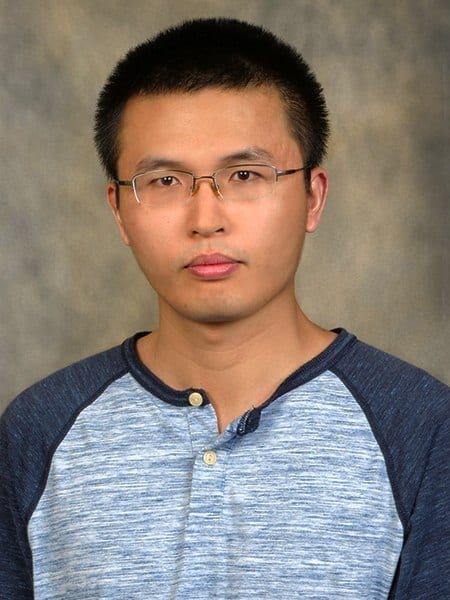
Faculty Mentor:
Dr. Yukun Li
His research revolves around computational finance. In the summer of 2024, REU students will be working on projects related to derivative pricing models based on the PDE approach and the data approach.
Project 4: Mathematical Biology
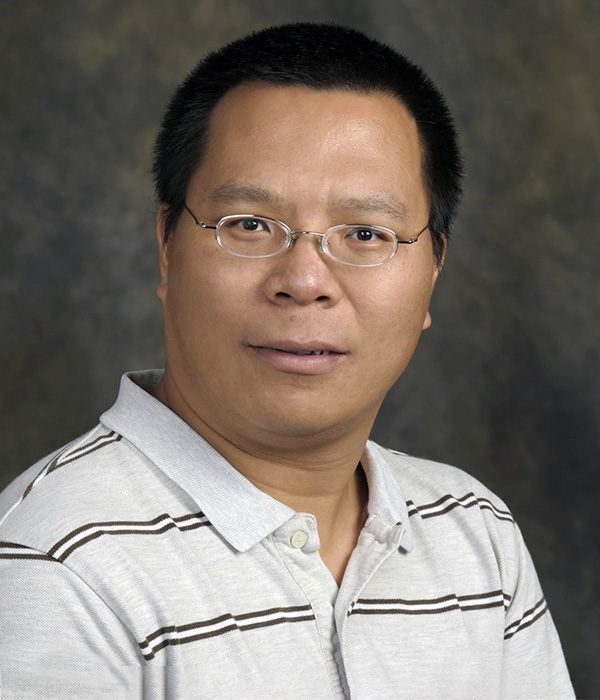
Faculty Mentor:
Dr. Zhisheng Shuai
His research focuses on mathematical ecology and epidemiology. In the summer of 2024, REU students will be working on projects related to mathematical modeling and control of infectious diseases.
Project 5: Applied Linear Algebra
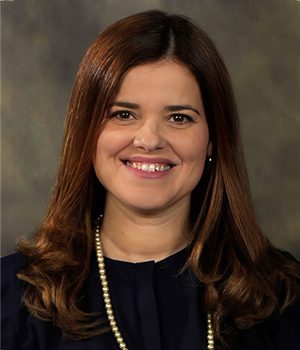
Faculty Mentor:
Dr. Katiuscia Teixeira
Her research revolves around the generation of knowledge on the processes of teaching and learning mathematics for STEM students. In the summer of 2024, REU students will be working on projects related to application of linear algebra.
2023 Participants & Projects
Project 1: Regularity Results for Convex Lagrangians in IRn
Faculty Mentor: Prof. Eduardo Teixeira
REU Students: Jacob Shaw – Clemson University
Ngoc Tram Nguyen – University of California, Los Angeles
Maximo Fernandez – University of Central Florida
Project 2: Curriculum Learning for Inverse Scattering
Faculty Mentor: Dr. Carlos Borges
REU Students: Mikayla Fischer – University of Central Florida
Nickolas Arustamyan – University of Florida
Megan Carlson – University of Central Florida
Project 3: PDE and Data Models for Derivative Pricing
Faculty Mentor: Dr. Yukun Li
REU Students: Anupama Hazarika – University of Arizona,
Alsion Tafa – University of Central Florida
Yiyuan Zhang – University of Florida
Project 4: Mathematical Approaches to Infectious Disease Control and Prevention
Faculty Mentor: Dr. Zhisheng Shuai
REU Students: Brendan Shrader – University of Central Florida
Malcolm Griffith – University of Central Florida
For more information or any questions please contact the Principle Investigator, Dr. Katiuscia Teixeira at Katiuscia.Teixeira@ucf.edu.